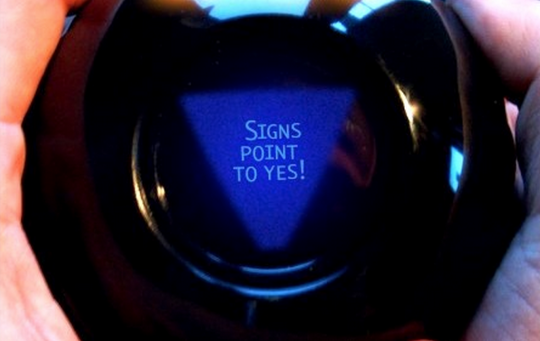
Are click through rates on search results a ranking signal? The idea is that if the third result on a page is clicked more often than the first that it will, over time, rise to the second or first result.
I remember this question being asked numerous times when I was just starting out in the industry. Google representatives employed a potent combination of tap dancing and hand waving when asked directly. They were so good at doing this that we stopped hounding them and over the last few years I rarely hear people talking about, let alone asking, this question.
Perhaps it’s because more and more people aren’t focused on the algorithm itself and are instead focused on developing sites, content and experiences that will be rewarded by the algorithm. That’s actually the right strategy. Yet I still believe it’s important to understand the algorithm and how it might impact your search efforts.
Following is an exploration of why I believe click-through rate is a ranking signal.
Occam’s Razor
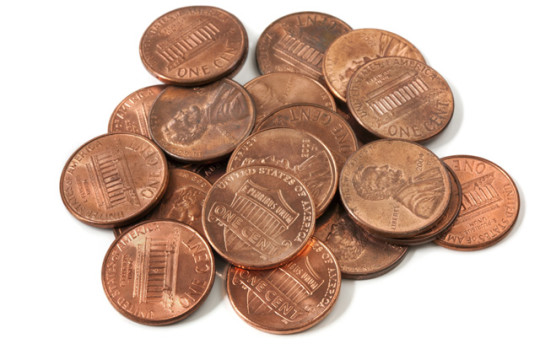
Though the original principle wasn’t as clear cut, today’s interpretation of Occam’s Razor is that the simplest answer is usually the correct one. So what’s more plausible? That Google uses click-through rate as a signal or that the most data driven company in the world would ignore direct measurement from their own product?
It just seems like common sense, doesn’t it? Of course, we humans are often wired to make poor assumptions. And don’t get me started on jumping to conclusions based on correlations.
The argument against is that even Google would have a devil of a time using click-through rate as a signal across the millions of results for a wide variety of queries. Their resources are finite and perhaps it’s just too hard to harness this valuable but noisy data.
The Horse’s Mouth
It gets more difficult to make the case against Google using click-through rate as a signal when you get confirmation right from the horse’s mouth.
That seems pretty close to a smoking gun doesn’t it?
Now, perhaps Google wants to play a game of semantics. Click-through rate isn’t a ranking signal. It’s a feedback signal. It just happens to be a feedback signal that influences rank!
Call it what you want, at the end of the day it sure sounds like click-through rate can impact rank.
[Updated 7/22/15]
Want more? I couldn’t find this quote the first time around but here’s Marissa Mayer in the FTC staff report (pdf) on antitrust allegations.
According to Marissa Mayer, Google did not use click-through rates to determine the position of the Universal Search properties because it would take too long to move up on the SERP on the basis of user click-through rate.
In other words, they ignored click data to ensure Google properties were slotted in the first position.
Then there’s former Google engineer Edmond Lau in an answer on Quora.
It’s pretty clear that any reasonable search engine would use click data on their own results to feed back into ranking to improve the quality of search results. Infrequently clicked results should drop toward the bottom because they’re less relevant, and frequently clicked results bubble toward the top. Building a feedback loop is a fairly obvious step forward in quality for both search and recommendations systems, and a smart search engine would incorporate the data.
So is Google a reasonable and smart search engine?
The Old Days
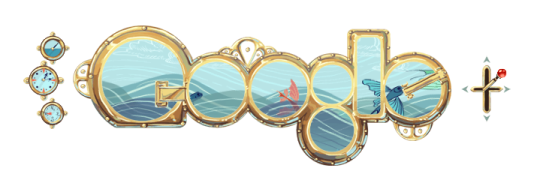
There are other indications that Google has the ability to monitor click activity on a query by query basis, and that they’ve had that capability for dog years.
Here’s an excerpt from a 2007 interview with Marissa Mayer, then VP of Search Products, on the implementation of the OneBox.
We hold them to a very high click through rate expectation and if they don’t meet that click through rate, the OneBox gets turned off on that particular query. We have an automated system that looks at click through rates per OneBox presentation per query. So it might be that news is performing really well on Bush today but it’s not performing very well on another term, it ultimately gets turned off due to lack of click through rates. We are authorizing it in a way that’s scalable and does a pretty good job enforcing relevance.
So way back in 2007 (eight years ago folks!) Google was able to create a scalable solution to using click-through rate per query to determine the display of a OneBox.
That seems to poke holes in the idea that Google doesn’t have the horsepower to use click-through rate as a signal.
The Bing Argument
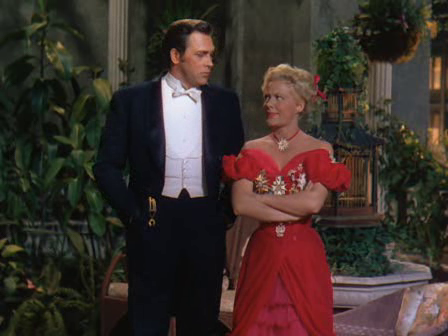
Others might argue that if Bing is using click-through rate as a signal that Google surely must be as well. Here’s what Duane Forrester, Senior Product Manager for Bing Webmaster Outreach (or something like that) said to Eric Enge in 2011.
We are looking to see if we show your result in a #1, does it get a click and does the user come back to us within a reasonable timeframe or do they come back almost instantly?
Do they come back and click on #2, and what’s their action with #2? Did they seem to be more pleased with #2 based on a number of factors or was it the same scenario as #1? Then, did they click on anything else?
We are watching the user’s behavior to understand which result we showed them seemed to be the most relevant in their opinion, and their opinion is voiced by their actions.
This and other conversations I’ve had make me confident that click-through rate is used as a ranking signal by Bing. The argument against is that Google is so far ahead of Bing that they may have tested and discarded click-through rate as a signal.
Yet as other evidence piles up, perhaps Google didn’t discard click-through rate but simply uses it more effectively.
Pogosticking and Long Clicks
Duane’s remarks also tease out a little bit more about how click-through rate would be used and applied. It’s not a metric used in isolation but measured in terms of time spent on that clicked result, whether they returned to the SERP and if they then refined their search or clicked on another result.
When you really think about it, if pogosticking and long clicks are real measures then click-through rate must be part of the equation. You can’t calculate the former metrics without having the click-through rate data.
And when you dig deeper Google does talk about ‘click data’ and ‘click signals’ quite a bit. So once again perhaps it’s all a game of semantics and the equivalent to Bill Clinton clarifying the meaning of ‘is’.
Seeing Is Believing
A handful of prominent SEOs have tested whether click-through rate influences rank. Rand Fishkin has been leading that charge for a number of years.
Back in May of 2014 he performed a test with some interesting results. But it was a long-tail term and other factors might have explained the behavior.
But just the other day he ran another version of the same test.
However, critics will point out that the result in question is once again at #4, indicating that click-through rate isn’t a ranking signal.
But clearly the burst of searches and clicks had some sort of effect, even if it was temporary, right? So might Google have developed mechanisms to combat this type of ‘bombing’ of click-through rate? Or perhaps the system identifies bursts in query and clicks and reacts to meet a real time or ‘fresh’ need?
Either way it shows that the click-through behavior is monitored. Combined with the admission from Udi Manber it seems like the click-through rate distribution has to be consistently off of the baseline for a material amount of time to impact rank.
In other words, all the testing in the world by a small band of SEOs is a drop in the ocean of the total click stream. So even if we can move the needle for a small time, the data self-corrects.
But Rand isn’t the only one testing this stuff. Darren Shaw has also experimented with this within the local SEO landscape.
Darren’s results aren’t fool proof either. You could argue that Google representatives within local might not be the most knowledgable about these things. But it certainly adds to a drumbeat of evidence that clicks matter.
But wait, there’s more. Much more.
Show Me The Patents
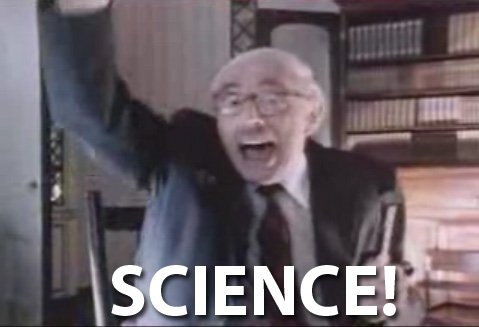
For quite a while I was conflicted about this topic because of one major stumbling block. You wouldn’t be able to develop a click-through rate model based on all the various types of displays on a result.
The result that had a review rich snippet gets a higher click-through rate because the eye gravitates to it. Google wouldn’t want to reward that result from a click-through rate perspective just because of the display.
Or what happens when the result has an image result or a answer box or video result or any number of different elements? There seemed to be too many variations to create a workable model.
But then I got hold of two Google patents titled Modifying search result ranking based on implicit user feedback and Modifying search result ranking based on implicit user feedback and a model of presentation bias.
The second patent seems to build from the first with the inventor in common being Hyung-Jin Kim.
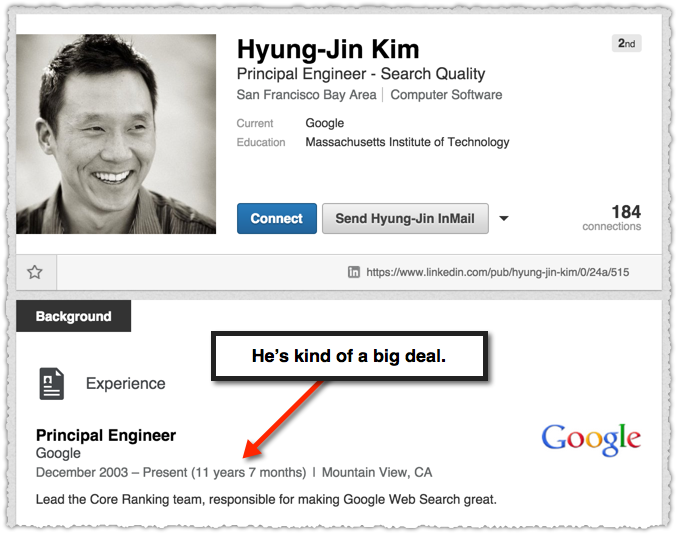
Both of these are rather dense patents and it reminds me that we should all thank Bill Slawski for his tireless work in reading and rendering patents more accessible to the community.
I’ll be quoting from both patents (there’s a tremendous amount of overlap) but here’s the initial bit that encouraged me to put the headphones on and focus on decoding the patent syntax.
The basic rationale embodied by this approach is that, if a result is expected to have a higher click rate due to presentation bias, this result’s click evidence should be discounted; and if the result is expected to have a lower click rate due to presentation bias, this result’s click evidence should be over-counted.
Very soon after this the patent goes on to detail the number of different types of presentation bias. So this essentially means that Google saw the same problem but figured out how to deal with presentation bias so that it could rely on ‘click evidence’.
Then there’s this rather nicely summarized 10,000 foot view of the issue.
In general, a wide range of information can be collected and used to modify or tune the click signal from the user to make the signal, and the future search results provided, a better fit for the user’s needs. Thus, user interactions with the rankings presented to the users of the information retrieval system can be used to improve future rankings.
Again, no one is saying that click-through rate can be used in isolation. But it clearly seems to be one way that Google has thought about re-ranking results.
But it gets better as you go further into these patents.
The information gathered for each click can include: (1) the query (Q) the user entered, (2) the document result (D) the user clicked on, (3) the time (T) on the document, (4) the interface language (L) (which can be given by the user), (5) the country (C) of the user (which can be identified by the host that they use, such as www-google-co-uk to indicate the United Kingdom), and (6) additional aspects of the user and session. The time (T) can be measured as the time between the initial click through to the document result until the time the user comes back to the main page and clicks on another document result. Moreover, an assessment can be made about the time (T) regarding whether this time indicates a longer view of the document result or a shorter view of the document result, since longer views are generally indicative of quality for the clicked through result. This assessment about the time (T) can further be made in conjunction with various weighting techniques.
Here we see clear references to how to measure long clicks and later on they even begin to use the ‘long clicks’ terminology. (In fact, there’s mention of long, medium and short clicks.)
But does it take into account different classes of queries? Sure does.
Traditional clustering techniques can also be used to identify the query categories. This can involve using generalized clustering algorithms to analyze historic queries based on features such as the broad nature of the query (e.g., informational or navigational), length of the query, and mean document staytime for the query. These types of features can be measured for historical queries, and the threshold(s) can be adjusted accordingly. For example, K means clustering can be performed on the average duration times for the observed queries, and the threshold(s) can be adjusted based on the resulting clusters.
This shows that Google may adjust what they view as a good click based on the type of query.
But what about types of users. That’s when it all goes to hell in a hand basket right? Nope. Google figured that out.
Moreover, the weighting can be adjusted based on the determined type of the user both in terms of how click duration is translated into good clicks versus not-so-good clicks, and in terms of how much weight to give to the good clicks from a particular user group versus another user group. Some user’s implicit feedback may be more valuable than other users due to the details of a user’s review process. For example, a user that almost always clicks on the highest ranked result can have his good clicks assigned lower weights than a user who more often clicks results lower in the ranking first (since the second user is likely more discriminating in his assessment of what constitutes a good result).
Users are not created equal and Google may weight the click data it receives accordingly.
But they’re missing the boat on topical expertise, right? Not so fast!
In addition, a user can be classified based on his or her query stream. Users that issue many queries on (or related to) a given topic (e.g., queries related to law) can be presumed to have a high degree of expertise with respect to the given topic, and their click data can be weighted accordingly for other queries by them on (or related to) the given topic.
Google may identify topical experts based on queries and weight their click data more heavily.
Frankly, it’s pretty amazing to read this stuff and see just how far Google has teased this out. In fact, they built in safeguards for the type of tests the industry conducts.
Note that safeguards against spammers (users who generate fraudulent clicks in an attempt to boost certain search results) can be taken to help ensure that the user selection data is meaningful, even when very little data is available for a given (rare) query. These safeguards can include employing a user model that describes how a user should behave over time, and if a user doesn’t conform to this model, their click data can be disregarded. The safeguards can be designed to accomplish two main objectives: (1) ensure democracy in the votes (e.g., one single vote per cookie and/or IP for a given query-URL pair), and (2) entirely remove the information coming from cookies or IP addresses that do not look natural in their browsing behavior (e.g., abnormal distribution of click positions, click durations, clicks_per_minute/hour/day, etc.). Suspicious clicks can be removed, and the click signals for queries that appear to be spammed need not be used (e.g., queries for which the clicks feature a distribution of user agents, cookie ages, etc. that do not look normal).
As I mentioned, I’m guessing the short-lived results of our tests are indicative of Google identifying and then ‘disregarding’ that click data. Not only that, they might decide that the cohort of users who engage in this behavior won’t be used (or their impact will be weighted less) in the future.
What this all leads up to is a rank modifier engine that uses implicit feedback (click data) to change search results.
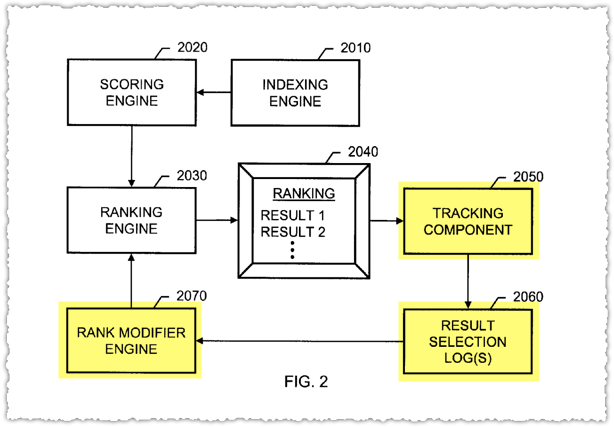
Here’s a fairly clear description from the patent.
A ranking sub-system can include a rank modifier engine that uses implicit user feedback to cause re-ranking of search results in order to improve the final ranking presented to a user of an information retrieval system.
It tracks and logs … everything and uses that to build a rank modifier engine that is then fed back into the ranking engine proper.
But, But, But
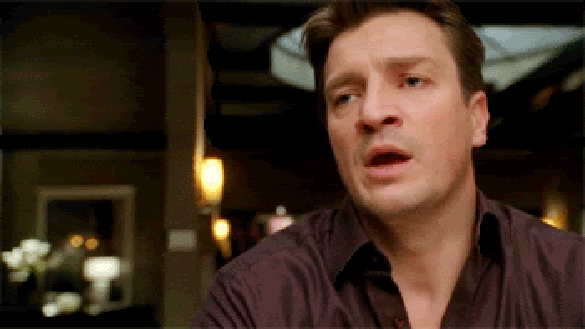
Of course this type of system would get tougher as more of the results were personalized. Yet, the way the data is collected seems to indicate that they could overcome this problem.
Google seems to know the inherent quality and relevance of a document, in fact of all documents returned on a SERP. As such they can apply and mitigate the individual user and presentation bias inherent in personalization.
And it’s personalization where Google admits click data is used. But they still deny that it’s used as a ranking signal.
Perhaps it’s a semantics game and if we asked if some combination of ‘click data’ was used to modify results they’d say yes. Or maybe the patent work never made it into production. That’s a possibility.
But looking at it all together and applying Occam’s Razor I tend to think the click-through rate is used as a ranking signal. I don’t think it’s a strong signal but it’s a signal none the less.
Why Does It Matter?
You might be asking, so freaking what? Even if you believe click-through rate is a ranking signal, I’ve demonstrated that manipulating it may be a fool’s errand.
The reason click-through rate matters is that you can influence it with changes to your title tag and meta description. Maybe it’s not enough to tip the scales but trying is better than not isn’t it?
Those ‘old school’ SEO fundamentals are still important.
Or you could go the opposite direction and build your brand equity through other channels to the point where users would seek out your brand in search results irrespective of position.
Over time, that type of behavior could lead to better search rankings.
TL;DR
The evidence suggests that Google does use click-through rate as a ranking signal. Or, more specifically, Google uses click data as an implicit form of feedback to re-rank and improve search results.
Despite their denials, common sense, Google testimony and interviews, industry testing and patents all lend credence to this conclusion.